Re-posted from my research group's blog at UO
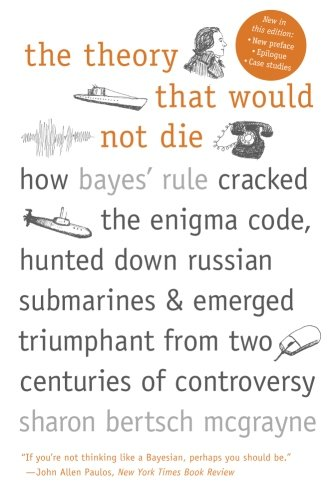
Now we see that frequentist and Bayesian statistics converge in the event of agnostic priors and lots of data: they are both valid ways of seeing the world. For rare events not easily controlled and replicated many times in a laboratory, Bayes is always superior. We update our beliefs all the time [citations not needed], but it becomes hard for people to own up to it and accept a quantifiable approach to this everyday action. No matter how many times people see Bayes rule, and no matter how much mathematical rigor connects it to the axioms of probability theory, people are always going to have trouble believing that you can do real science starting from "a hunch".
McGrayne dives into the early history, when factions fought each other with religious zeal and carried a full train of philosophical baggage along with their statistics. It was more than a way of using data, statistics was a worldview, and carried with it diametrically opposed ways of doing science. The impacts became real: addressing systemic injustice, identifying the causes of cancer, winning the world war, averting nuclear disaster.
Many of these problems also make for really cool worked examples to teach Bayes rule. Here are some of the highlights:
-Laplace
Really it should be called Laplace's rule, since he was the first to flesh it out in complete mathematical rigor. The world has settled on Bayes, figuring that Laplace had enough stuff named after him allready. He used it to nail down the orbits of planets, assigning the appropriate weight to observations from ancient Arab and Roman astronomers.Laplace offered a bet: "11,000 to 1 odds that Bouvard's results for Saturn were within 1% of the correct answer, and a million to one odds for Jupiter. Nobody seems to have taken Laplace's bet, but today's technology confirms that Laplace should have won both bets."
Laplace also managed to show conclusively that the birth rate for is higher for boys than girls in France. He exposed the injustices of the french legal system, showing that juries were rigged and the 'priors' on guilt were too high. By this time he commanded enough political weight that drastic changes were effected after his studies. Possibly due to his public service, Laplace managed to survive the french revolution with head and neck fully intact, despite being a nobleman. This is a high honor.
-Turing and the enigma code.
Banburismus was the Bayesian process that Alan Turing developed to crack the Nazi enigma code. The allies acknowledged that this breakthrough may have shortened the war in Europe by 2-4 years.He invented a new unit of probability, the Ban, which was intended to represent the smallest quanta of chance, or unit of weight of evidence favoring a hypothesis, that humans can reliably distinguish.
As well as being the father of modern computing and AI, Turing was a Bayesian of sorts. Unfortunately, he couldn't share any of his work at Bletchley Park with the scientific community at large, since it remained classified for decades after the war. To this day many people don't know just how groundbreaking his work was in many disparate fields from biology to cryptography.
-Smoking causes cancer.
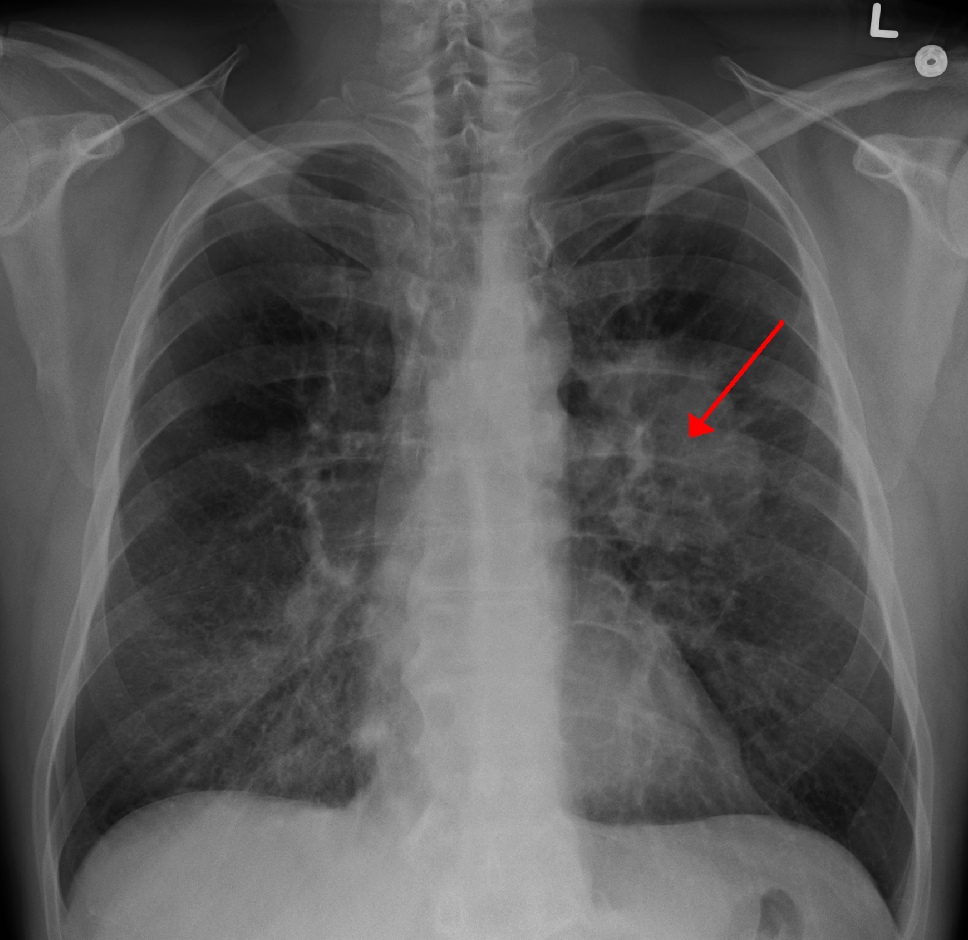
-Federalist Papers
-Clinical Trials
When trying to bring a new therapy out of development and into use, following the doctrines of frequentist statistics can require orders of magnitude more participants and trials than an equivalent Bayesian analysis, all to come to a similar conclusion about the efficacy. This becomes a humanitarian issue if people are suffering from a serious disease and being given placebos or ineffective treatments in large numbers. Bayesian analysis of clinical results makes this convoluted process more effective.-Diagnosis
In medicine, diagnosis is rife with uncertainty. For doctors, the only certainties are the symptoms: what can be learned from studying the patient. The entirety of medical literature describes conditions, diseases, injuries, etc. and then secondarily includes the symptoms and maybe some documented chance of that symptom being present. Diagnosis can be approached as an inverse probability problem that is amenable to Bayes rule: Given the following symptoms, what is the probability that you have measles, mumps, a pulmonary embolism, whatever. Doctors need to know the statistics, but they still frequently make diagnoses intuitively. It's common these days for computer diagnosis to outperform expert diagnosis, and many of these systems are built on a foundation of Bayes.-Whale population estimates.
The ocean is vast [citation needed], and whales, despite also being big[citation needed], are hard to track and count. They surface only occasionally and range over huge areas. Constant, accurate monitoring of their populations is prohibitively difficult. The goal is really just to see whether the population is declining or thriving, and to see if changes in policy are having an effect. So, we have completely inadequate, sketchy data, but we need to make important decisions that could affect the future of entire species. This is a perfect job for Bayes. A similar approach is now applied to the study of many threatened or endangered species, and in quantitative ecology more broadly.-The case of the missing H-Bombs
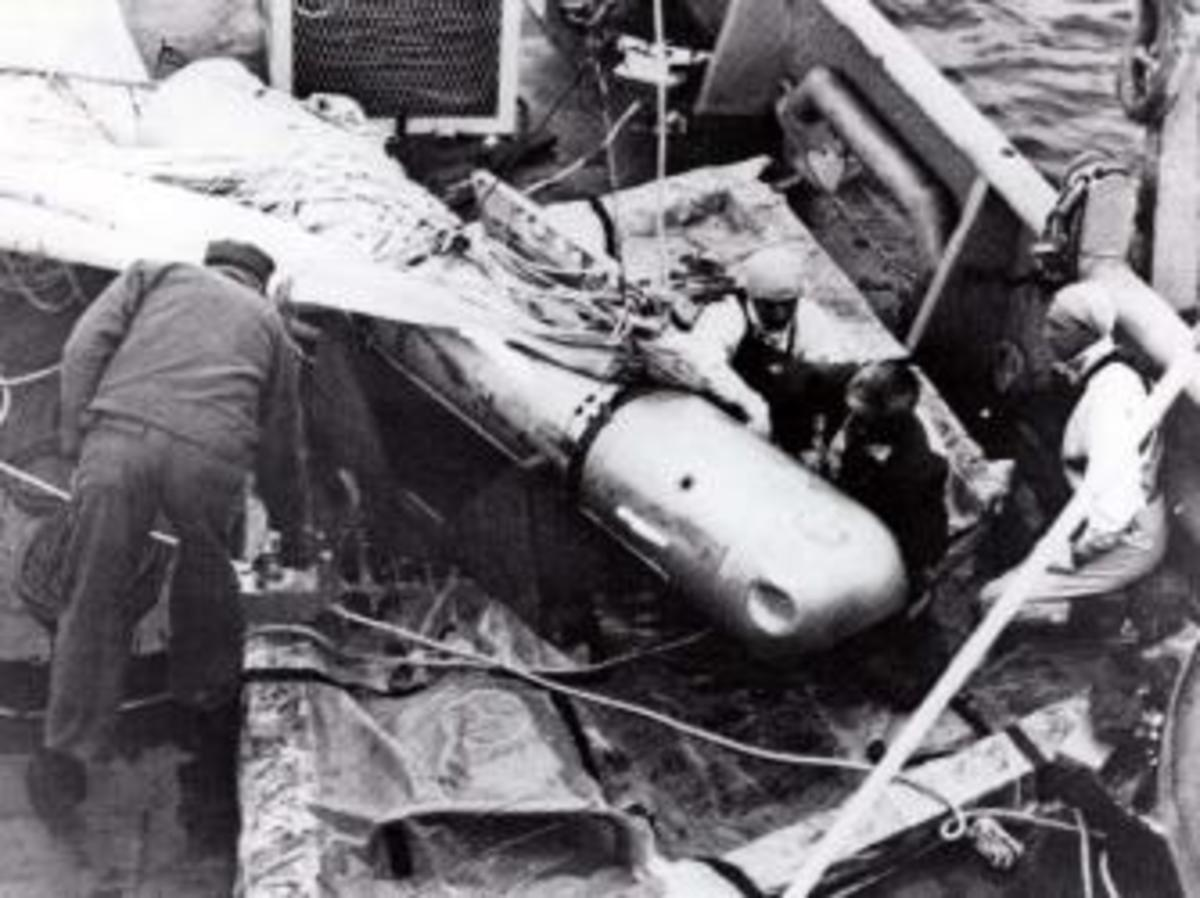
-Actuaries
When science repeatedly abandoned Bayes rule, actuaries kept it alive every time, humming away in the boring but rather important insurance industry. Under the guise of "credibility theory", they optimized their insurance premiums in a world with highly uncertain but quantifiable risks. It made sense to update belief in the insurance business as accidents, claims, and deeper statistics accumulated and people sharpened their insurance game. To invest in shared risk and make it profitable it's important to be right most of the time(like how the house usually wins at blackjack). While they didn't officially acknowledge it as such, the industry updated their tables each year for many decades with a modified version of Bayes rule, developed independently for their purposes.policing and racial profiling lindley
-Challenger Disaster.
A Bayesian analysis of the O-ring failure. Numerous examples of this calculation can be found online. Tracing the culpability to this part was a problem rife with uncertainty, and ended up being a job for Bayes.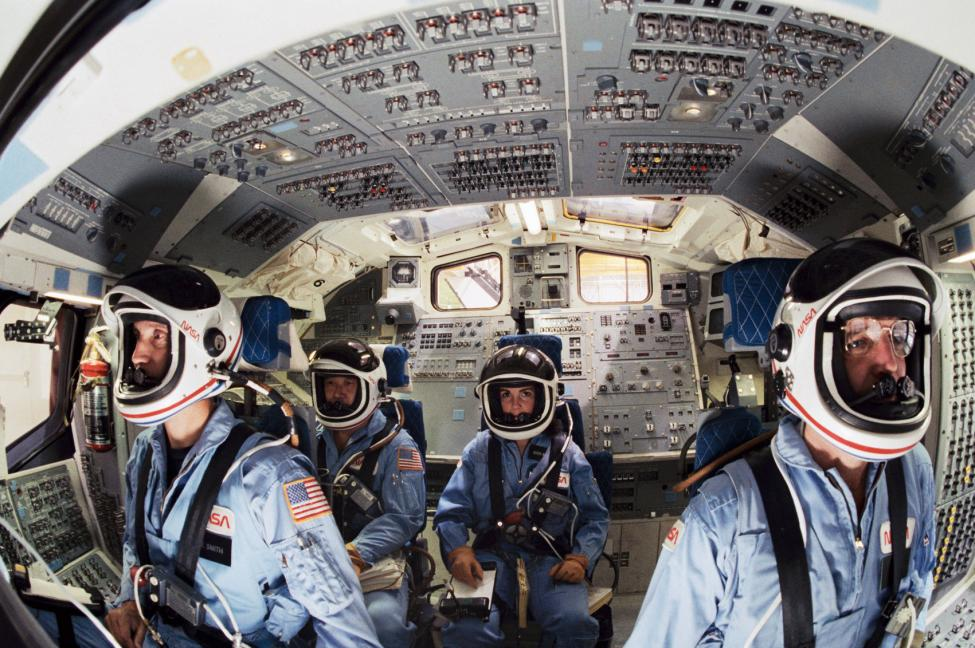
-Clippy the paperclip.
I'm young, but not so young that I don't remember Microsoft Word's infamous office assistant, Clippy (2003-2007). RIP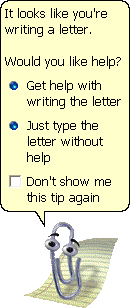
-Nate Silver at 538
In the 2008 election Nate Silver correctly predicted the outcome in 49 states with a novel Bayesian algorithm. This was a previously unmatched level of performance in predictive political science. He became a real household name in America, and likely the most publicly famous statistician of the 21st century thus far. Despite being tragically wrong about the 2016 election (blerg), his methods are pretty interesting.-google translate
Uses Bayes. Who knew?-monkeys: robotic arm control
The ultimate goal: to allow amputees to control robotic arms with only their neural impulses.Electrodes were implanted in the motor and premotor cortices of Rhesius monkeys, and then successfully used to control robotic arms. The monkeys were able to perform accurate movements to achieve specific goals, like grabbing treats. The huge challenge here is turning neural signals, which often appear to be stochastic, into coherent movements.
theoretical neuro, waldport
monk prior joke.
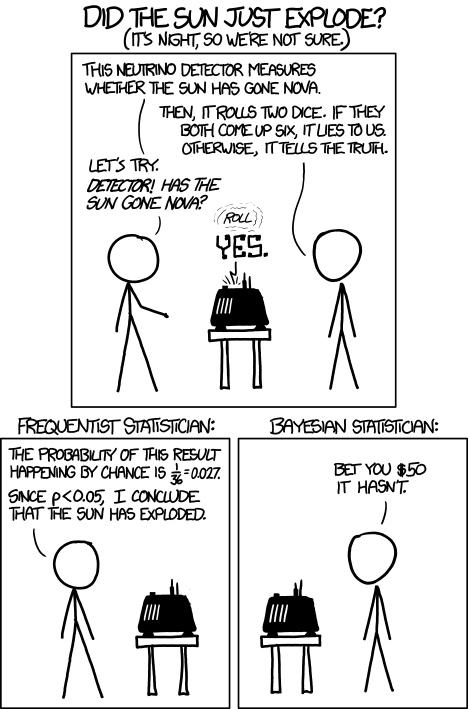
Refs:
[1] Sharon Bertsch McGrayne, The Theory that would not die...[2] lukeprog, A History of Bayes Theorem, https://www.lesswrong.com/posts/RTt59BtFLqQbsSiqd/a-history-of-bayes-theorem ***note, a really fun comments section on this one...
[3] Randall Monroe, https://xkcd.com/1132/
[4] https://www.google.com/search?sxsrf=ACYBGNQ3IqoKYkGdRvXV6AQFzP7p20cs2Q:1578456995531&q=The+Signal+and+the+Noise&stick=H4sIAAAAAAAAAONgFuLSz9U3KCuJT09JUQKz80zK07PKtMSyk630k_Lzs8GEVXFmSmp5YmXxKkbJNKvi0qSs1OQSK5BMsRVIk5FlWlH6IlaJkIxUheDM9LzEHIXEvBSFEiDXLz-zOBUAdNMTImkAAAA&sa=X&ved=2ahUKEwjM7ovEkvPmAhWtIjQIHSWeCt0QxA0wFnoECBUQDQ&sxsrf=ACYBGNQ3IqoKYkGdRvXV6AQFzP7p20cs2Q:1578456995531&biw=2357&bih=1857
[5] https://en.wikipedia.org/wiki/Office_Assistant#cite_note-AlanCooper-8
No comments:
Post a Comment